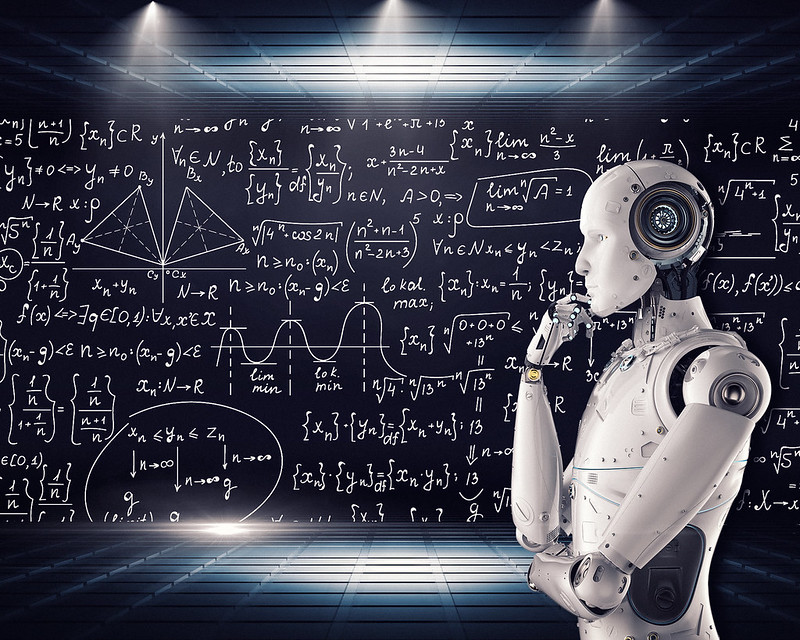
Examining Algorithms’ Effects on Online Biases
By: Henry Rademacher
On November 12, the Brookings Institution Center for Technology and Innovation hosted a panel on online bias.
The event was the second in a series of panel discussions Brookings is hosting on bias in artificial intelligence (AI), an issue that has garnered increased publicity as AI becomes more and more prevalent in everyday life. Studies show that many AI systems currently in use tend to show bias, most commonly against women and people of color.
The panel began by discussing a recent scandal, in which the algorithm used to assign credit to users of the new Apple Card gave substantially more credit to men than women. Dr. Karl Ricanek, a professor of computer science at the University of North Carolina Wilmington, described this as “fundamentally a flaw,” explaining that machine learning algorithms “tend to pick up on characteristics that may be implied or inferred in some roundabout way.”
According to Dr. Ricanek, these algorithms are able “to discover facts and then apply them in negative ways” even though their designers do not intend for this to happen.
These biases impact many aspects of people’s lives, as illustrated by a recent case of a widely-used health care prediction algorithm displaying substantial bias against black patients. Natasha Duarte, a policy analyst at the Center for Democracy and Technology, attributed this to the fact that algorithms can rely on “features that are disproportionately distributed” between populations, for example the amount that black Americans spend on health care compared to white Americans.
The biases in health care algorithms are especially problematic because they affect so many people.
But solving these issues is difficult, particularly because there is a lack of publicly available information on how AI algorithms work. Dr. Solon Barocas, a professor in the Department of Information Science at Cornell University, added that this lack of information causes people to “speculate wildly” on what is wrong with the systems.
Dr. Barocas also pointed out that many individuals may experience bias at the hands of algorithms without having any awareness of it happening because an individual is unlikely to “see the aggregate effect” of a decision made by an algorithmic system.
The Brookings Institution’s Dr. Nicol Turner Lee concurred with Dr. Barocas, pointing out that affinity groups “largely define who you are” and that filter bubbles, themselves algorithms, can result in various affinity groups seeing or not seeing much of the same information.
Finally, the panel discussed bias in facial recognition, one of the issues that has caused the most alarm among both consumers and public policy advocates. Dr. Ricanek, who has worked with facial recognition software since the 1990s, emphasized that many developers “do not do the due diligence to understand the capacity of their systems to be biased.” He pointed out that 99 percent of the algorithms are based on some form of deep learning, meaning that many of these algorithms are capable of picking up on information that they were not necessarily programmed to use in decision making.
The use of facial recognition software by law enforcement has been especially controversial due to its apparent propensity to discriminate against black people. But Duarte pointed out that it’s not just the technology that is problematic. In her view, “there is a lot of misuse of the tools in the on-the-ground law enforcement settings.” This could be because the technology is new and evolving rapidly, while the officers may not have a background in technology.
All panelists agreed that it is difficult to identify solutions to the bias problems present in AI algorithms. The issue is further complicated by policymakers’ differing approaches.
“Some people want to advocate for improving these systems, some people want to advocate for banning them,” Dr. Baroca said.
Photo credit: Mike MacKenzie (flickr)